Zoekcriteria
Vind jouw baan in onze 138 vacatures!
Raalte
Teamleider havo bovenbouw (Carmel College Salland, Raalte)
Ben jij een leider die het team meeneemt naar nieuwe hoogtes, met een glimlach, scherpe inzichten, daadkracht en een...
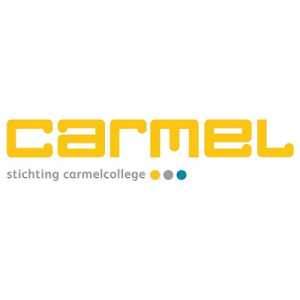
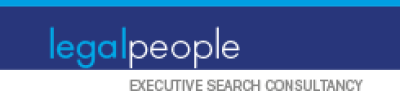
Losser
Adjunct-directeur B
Ben jij een stevige en inspirerende leider met oog voor mensen én onderwijs? Lees dan snel verder!
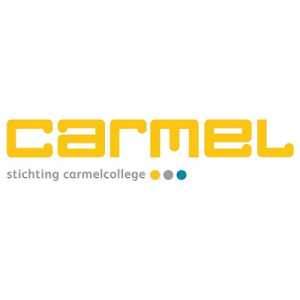
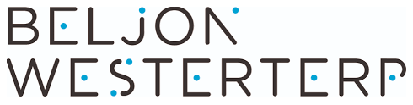
Manager Financiën en control
Leeuwendaal zoekt voor SEIN een bevlogen en verbindende manager Financiën en Control De organisatie...
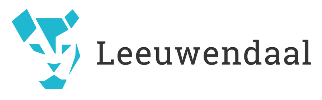
regio Utrecht
Accountmanager Regio Woerden, Amersfoort, Tiel, Ede
De Sales van STILL in Nederland bestaat uit een aantal regionaal verdeelde Accountmanagers. Deze vacature is voor de...
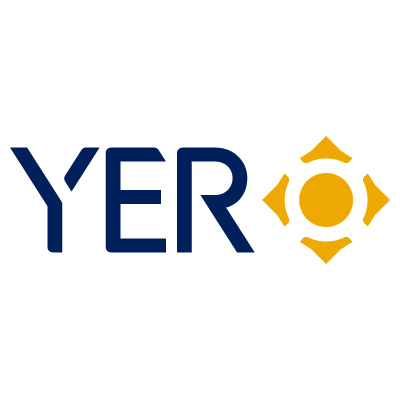
Haarlem
Supply Chain Specialist
Als Supply Chain Specialist ben je verantwoordelijk voor het coördineren van de internationale supply chain van...
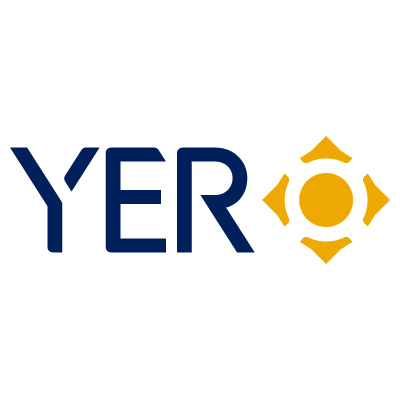
Regio Eindhoven
Product Manager / Application Support
As Product Manager, you serve as the key interface between Product Management and the R&D-, Product Development-,...
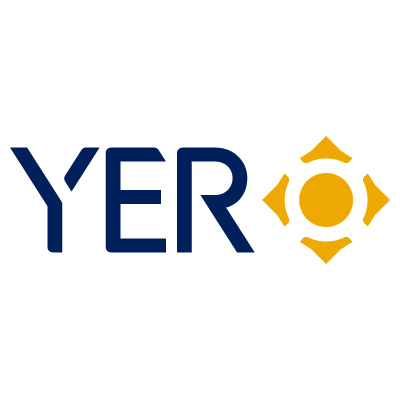
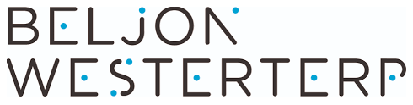
Enschede
Directeur Kottenpark
Ben jij een leider die koersvast is, maar ook ruimte biedt? Lees snel verder!
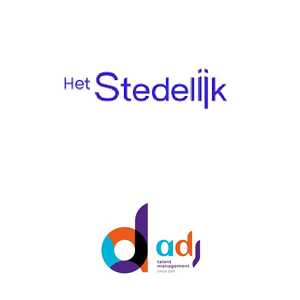
Schiedam
Senior Inkoper
Jouw expertise maakt impact! Wil jij je als Senior Inkoper inzetten om bij te dragen aan een gezonde, veilige en...
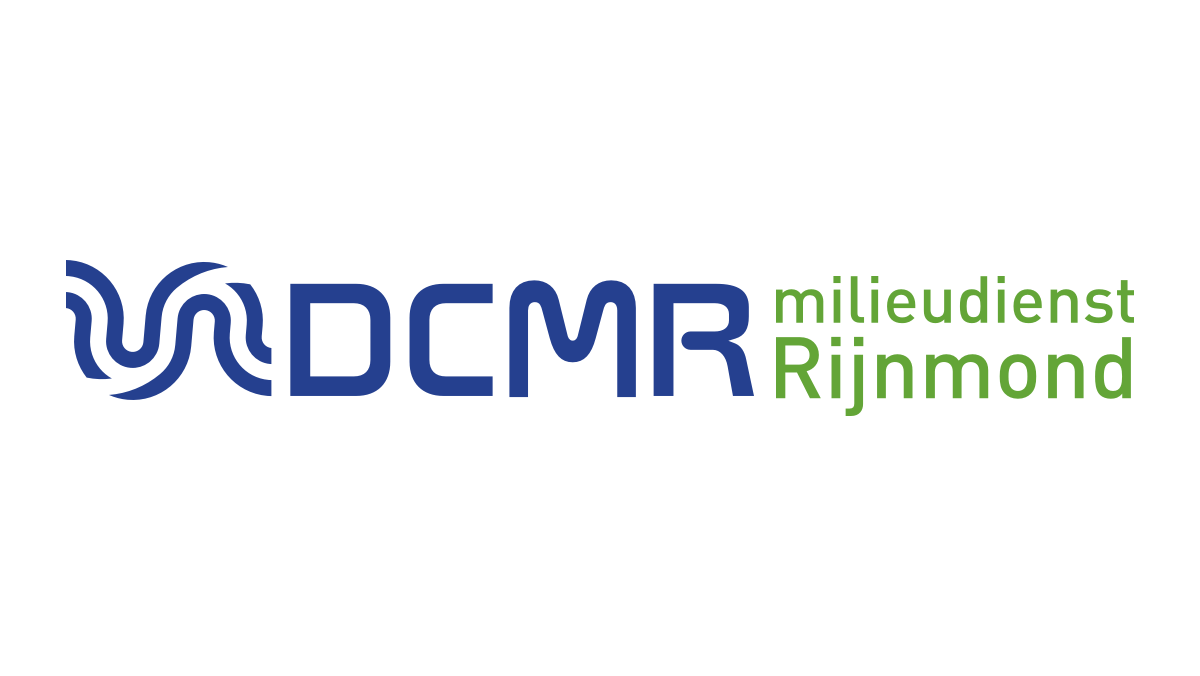
Doetinchem
Directiesecretaris
Ben jij een strategisch denker met scherp inzicht in bestuurlijke processen en organisatieontwikkeling? Als...
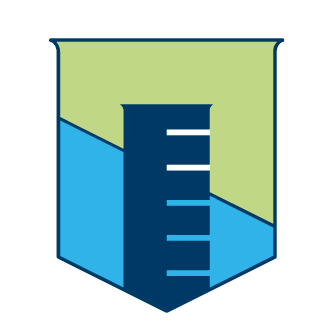
Spijkenisse
Lid Raad van Commissarissen profiel financiën en control
Boeiende nevenfunctie voor ervaren teamspeler met kennis van zaken en een brede bestuurlijke blik. Sociale...
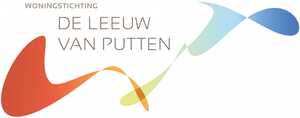
5242 CN
Manager Gezondheid en Kwaliteit
In deze rol krijg je de kans om jouw leiderschapsvaardigheden verder te ontwikkelen en een blijvende impact te maken...
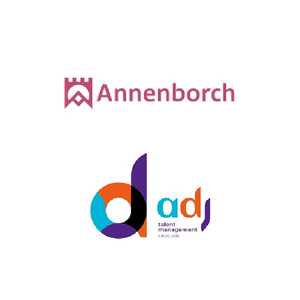
Den Haag
Strategisch adviseur regionale economie (24-36 uur)
Breng provincies samen, vertaal complexe economische ontwikkelingen naar strategie én impact, en bouw mee aan...
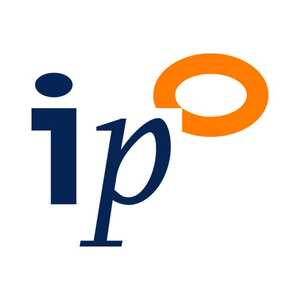
Haarlem
Beleidsadviseur Kabinet CdK
Wij zijn op zoek naar een Beleidsadviseur Kabinet CdK 32-36 uur Wil je werken in het hart van het provinciaal...
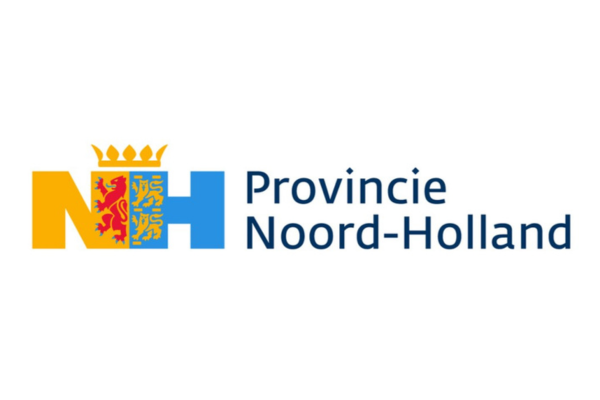
Doetinchem
Programmamanager Klimaat & Leefomgeving
Kun jij complexe maatschappelijke opgaven zoals een gezonde leefomgeving, een veilig gebied en klimaatneutraliteit...
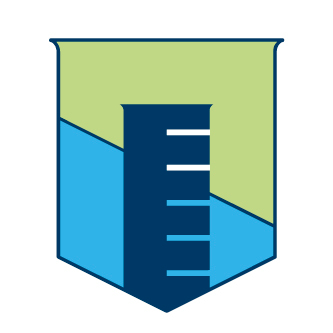
Schiedam
Directeur
Ben jij een gedreven directeur die het beste binnen de organisatie en het management naar boven haalt? Lees dan snel...
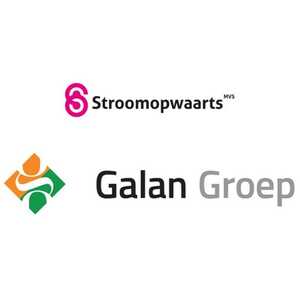
’s-Hertogenbosch
Hoofd Personeel & Organisatie en Facilitair
Geef strategisch leiderschap aan organisatieontwikkeling en breng mensen, processen en visie samen in een complexe en...
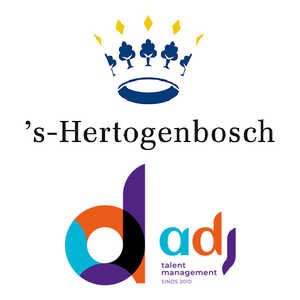
Utrecht
Bestuurssecretaris
Ben jij in staat om als bestuurssecretaris te zorgen voor stabiliteit en rust in een dynamische zorgorganisatie? Lees...
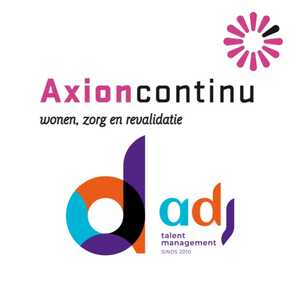
Sint Nicolaasga
Voorzitter en lid Raad van Toezicht
Samenwerking, netwerken, ontwikkeling en transitie
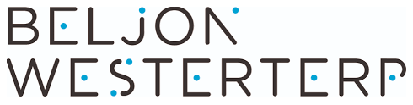
Hilversum
Voorzitter Raad van Toezicht (via PublicSpirit)
De NLPO zoekt een verbindende voorzitter, met politiek-bestuurlijk profiel en met gevoel voor de lokale omroep.
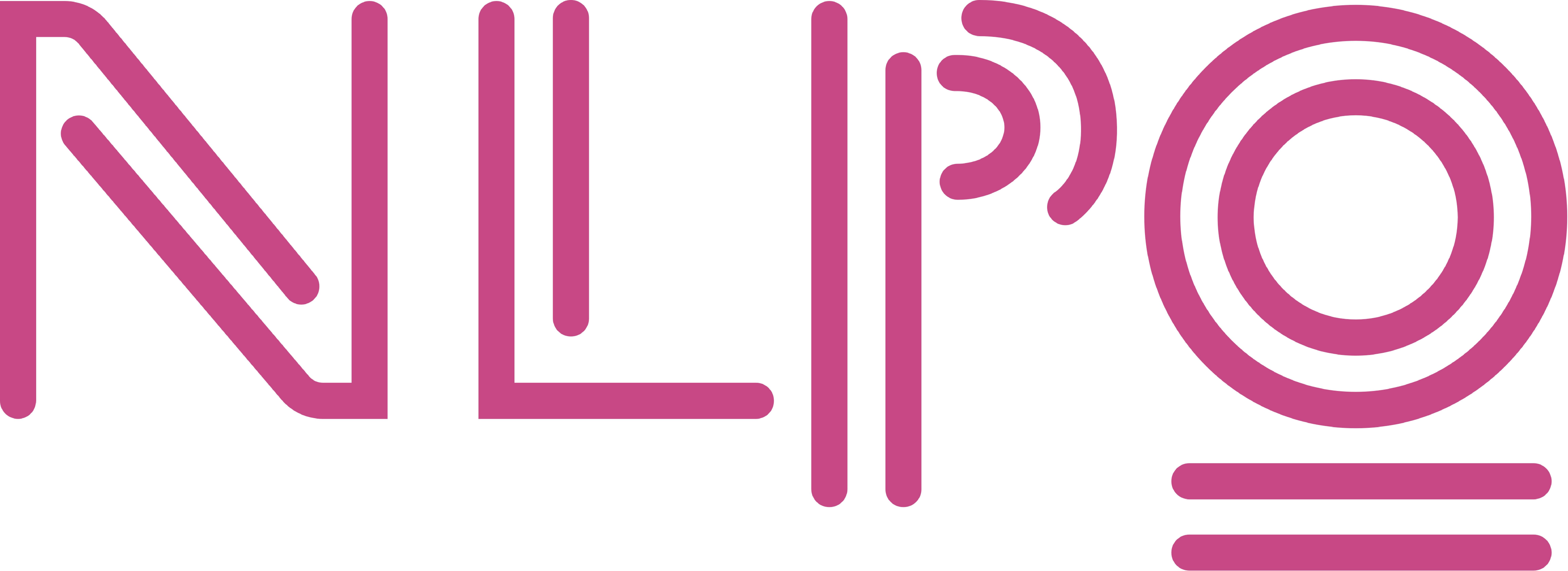
Utrecht
Lid Raad van Commissarissen (Lid RvC)
Voor Schola Medica is ADJ op zoek naar een enthousiast en collegiaal Lid voor de Raad van Commissarissen (Lid RvC)
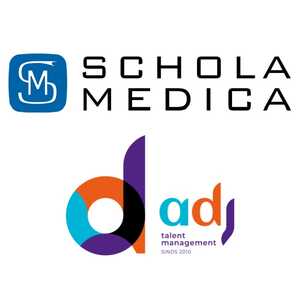
Rotterdam
Directeur-bestuurder
Een koersvaste directeur-bestuurder die rust en richting brengt en tegelijkertijd oog heeft voor de mensen met wie en...
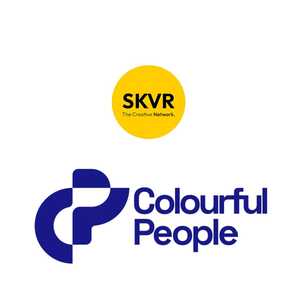
Alkmaar
Bestuurder
Leeuwendaal zoekt voor de csg Jan Arentsz een koersvaste, verbindende, strategische, innovatieve en inspirerende...
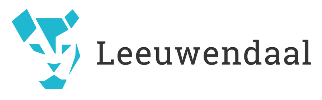
Haarlem
Financieel adviseur
Financieel adviseur Gevarieerd, uitdagend en betekenisvol. Speel een cruciale rol in het financiële beleid...
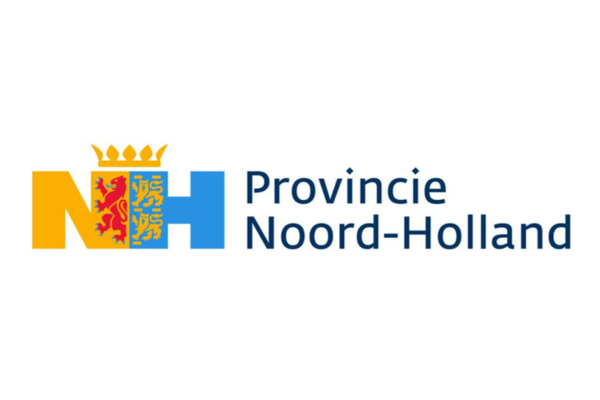